Navigating the complex terrain of modern data management requires a strategic approach to selecting the most suitable data architecture. With the exponential growth of data volumes and diversity, organizations face the daunting task of identifying architectures that align with their evolving business objectives.
Visual Flow’s expertise extends beyond mere guidance; their hands-on support ensures a smooth transition between data architectures, minimizing disruptions and maximizing the value derived from data assets. In the landscape of data management, choosing the right data architecture is crucial for organizations seeking to harness the full potential of their data assets.
Visual Flow, a trusted provider of innovative solutions for IT developers, offers a comprehensive guide to understanding the differences between data lakes and data warehouses and selecting the appropriate data architecture for specific business needs.
As a data migration services company, Visual Flow also assists organizations in seamlessly transitioning their data between different architectures, ensuring efficiency, integrity, and optimal performance throughout the process.
Understanding Data Lakes and Data Warehouses: Key Differentiators
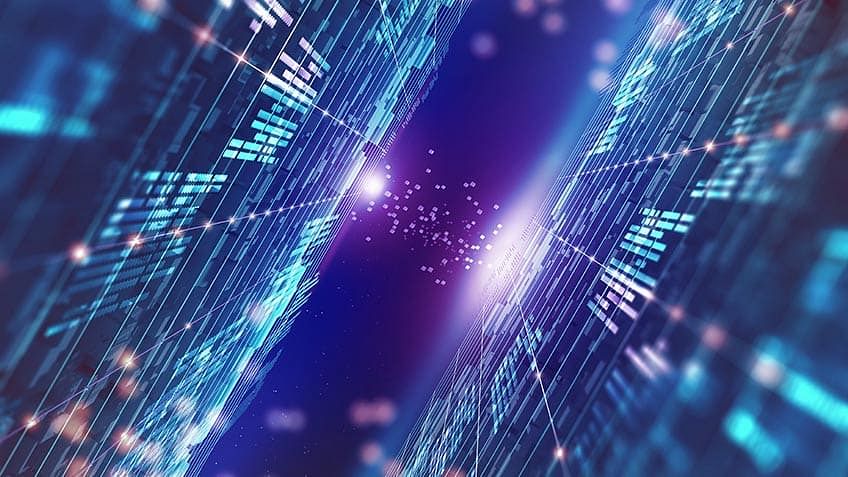
- Data Lakes: Data lakes are centralized repositories that store vast amounts of raw, unstructured, and semi-structured data in its native format.
- Data Warehouses: Data warehouses, on the other hand, are structured repositories that store processed, cleaned, and curated data optimized for analysis and reporting.
- Schema Flexibility: Data lakes offer schema-on-read flexibility, allowing data to be ingested without predefined schema, whereas data warehouses enforce schema-on-write, requiring data to conform to predefined structures.
- Data Types: Data lakes accommodate diverse data types, including text, images, and sensor data, while data warehouses primarily store structured transactional data from operational systems.
- Processing Paradigm: Data lakes support batch, real-time, and interactive processing paradigms, making them suitable for a wide range of analytical and exploratory use cases, whereas data warehouses are optimized for batch processing and complex analytical queries.
Use Cases for Data Lakes and Data Warehouses
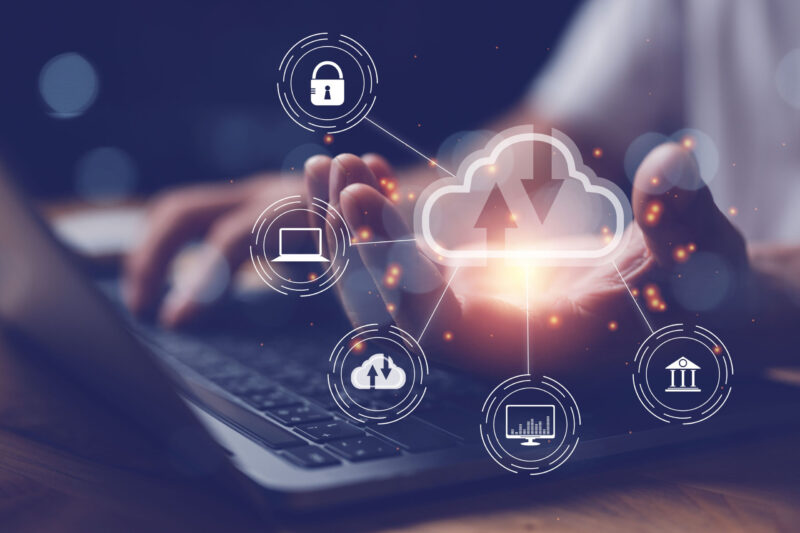
- Exploratory Analysis and Data Science: Data lakes are well-suited for exploratory analysis and data science projects, where the flexibility to ingest and analyze diverse datasets is essential.
- Big Data Processing: Data lakes excel in handling large volumes of data from various sources, making them ideal for big data processing and analytics.
- Raw Data Storage: Data lakes serve as a repository for raw, unstructured data, enabling organizations to retain data for future analysis and discovery.
- Operational Reporting and Business Intelligence: Data warehouses are optimized for operational reporting and business intelligence, providing fast query performance and predictable response times.
- Regulatory Compliance: Data warehouses are often preferred for regulatory compliance and governance purposes, as they enforce data integrity, consistency, and security measures.
- Structured Analytics: Data warehouses are suitable for structured analytics use cases, such as financial reporting, dashboarding, and executive analytics, where data quality and accuracy are paramount.
Considerations for Choosing the Right Data Architecture
- Data Variety: Assess the variety and complexity of data sources and types within the organization to determine the suitability of data lakes versus data warehouses.
- Analytical Requirements: Evaluate the analytical requirements, including query performance, latency, and scalability, to identify the most appropriate data architecture.
- Data Governance: Consider data governance and compliance requirements, such as data lineage, security, and privacy, when selecting the data architecture.
- Cost and Scalability: Compare the cost and scalability of data lakes and data warehouses, including infrastructure, storage, and operational expenses.
- Organizational Culture: Take into account the organizational culture, skill sets, and preferences of data stakeholders, as well as existing infrastructure and technology investments.
Integration with Cloud Services: Unlocking Scalability and Flexibility
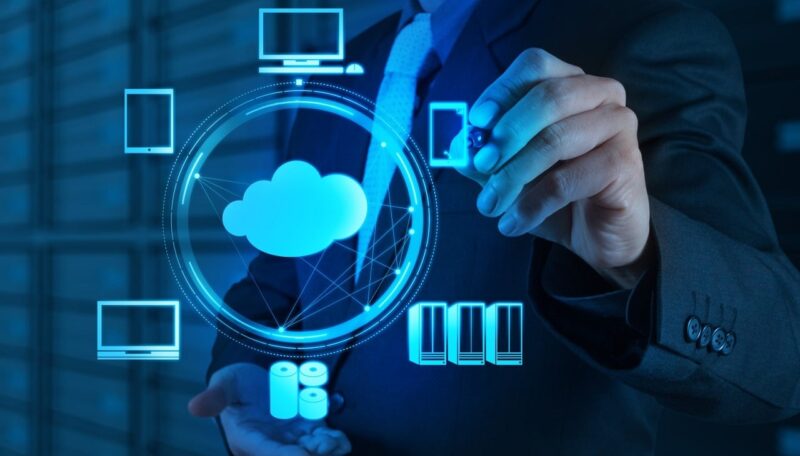
As organizations increasingly embrace cloud computing, the integration capabilities of data lakes and data warehouses with cloud services become paramount considerations. Data lakes, with their inherent scalability and flexibility, seamlessly integrate with various cloud platforms, enabling organizations to leverage cloud-native services for data storage, processing, and analytics.
This integration empowers businesses to adapt to changing data demands and scale their operations efficiently. Conversely, while data warehouses can also integrate with cloud environments, their structured nature may pose challenges in fully exploiting the elasticity and cost-effectiveness of cloud resources.
Therefore, organizations evaluating data architectures must assess the compatibility and integration capabilities with their chosen cloud infrastructure to maximize scalability and flexibility while minimizing operational overhead.
Real-time Analytics and Decision-making: Leveraging Data Lakes’ Agility
In today’s fast-paced business environment, real-time analytics and decision-making have become imperative for gaining competitive advantages. Data lakes offer significant advantages in this regard, providing the agility and responsiveness required to analyze streaming data and derive actionable insights instantaneously.
With support for real-time processing paradigms, such as stream processing frameworks and event-driven architectures, data lakes enable organizations to monitor, analyze, and respond to dynamic data streams in near real-time. This capability is particularly valuable in industries such as finance, e-commerce, and IoT, where timely insights drive strategic decision-making and operational efficiency.
In contrast, while data warehouses can handle real-time data to some extent, their batch-oriented processing model may introduce latency and hinder responsiveness in rapidly evolving scenarios. Therefore, organizations prioritizing real-time analytics should favor data lake architectures for their agility and ability to support continuous data ingestion and analysis.
Data Quality and Consistency: Ensuring Accuracy and Reliability
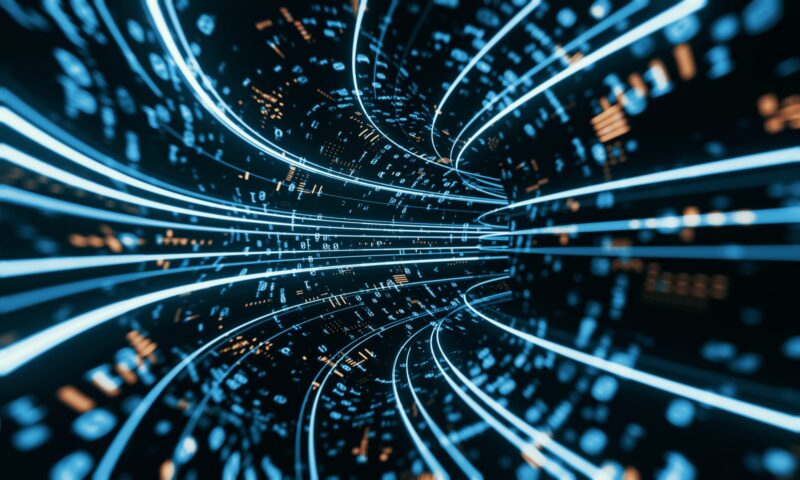
The quality and consistency of data are paramount concerns for organizations reliant on data-driven decision-making processes. Data warehouses excel in maintaining high data quality and consistency through rigorous schema enforcement, data cleansing, and validation processes. By adhering to predefined schemas and data integrity constraints, data warehouses ensure that analytical outputs are accurate, reliable, and consistent across different business functions.
Additionally, data governance frameworks integrated with data warehouses facilitate proactive data stewardship, lineage tracking, and auditing, further enhancing data quality and compliance. Conversely, data lakes, with their schema-on-read approach and lax governance mechanisms, may encounter challenges related to data quality assurance and consistency.
Without robust data governance practices and data quality management frameworks in place, organizations risk encountering discrepancies, inaccuracies, and inconsistencies in their analytical outputs.
Therefore, while data lakes offer unparalleled flexibility and scalability, organizations must implement stringent data quality measures and governance protocols to maintain the accuracy and reliability of their data assets.
Related Posts:
- The Role of Aluminum Windows: How They Contribute to…
- Choosing The Right Ninja Blender For Your Kitchen in 2024
- 5 Tips for Choosing the Right Variance When Playing…
- Choosing the Right Domain Hosting ─ Key Factors to…
- 10 Tips for Choosing the Perfect Gift for Your Loved…
- Common Mistakes Beginners Make When Choosing an…